Text embeddings#
A text embedding is a high-dimensional latent space where words or phrases are represented as vectors. In this notebook we will determine the vectors for a couple of words. For visualization purposes, we will apply principal-component-analysis to these vectors and display the relationship of the words in two-dimensional space.
import openai
import numpy as np
import matplotlib.pyplot as plt
from sklearn.decomposition import PCA
This helper functions will serve us to determine the vector for each word / text.
from llama_index.embeddings.huggingface import HuggingFaceEmbedding
embed_model = HuggingFaceEmbedding(model_name="BAAI/bge-base-en-v1.5")
def embed(text):
return embed_model._get_text_embedding(text)
vector = embed("Hello world")
vector[:3]
[0.010724308900535107, 0.05578264966607094, 0.027084074914455414]
len(vector)
768
words = ["dog", "cat", "hamster", "guinea pig", "pet", "microscope"]
# Example of input dictionary
object_coords = {word: embed(word) for word in words}
# Extract names and numerical lists
names = list(object_coords.keys())
data_matrix = np.array(list(object_coords.values()))
# Apply PCA
pca = PCA(n_components=2) # Reduce to 2 components for visualization
transformed_data = pca.fit_transform(data_matrix)
# Create scatter plot
plt.figure(figsize=(3, 3))
plt.scatter(transformed_data[:, 0], transformed_data[:, 1])
# Annotate data points with names
for i, name in enumerate(names):
plt.annotate(name, (transformed_data[i, 0], transformed_data[i, 1]))
plt.title('PCA of word embedding')
plt.xlabel('Principal Component 1')
plt.ylabel('Principal Component 2')
plt.show()
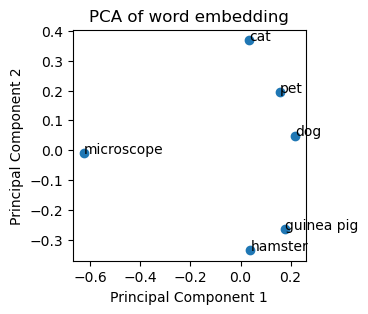
Exercise#
Draw an embedding for words such as “segmentation”, “thresholding”, “filtering”, “convolution”, “denoising”, “measuring”, “plotting”.
Could you predict how the words are placed in this space?
Draw the same embedding again - is the visualization repeatable?
Change the order of the words in the list. Would you expect the visualization to change?
Add words such as “banana”, “apple”, “orange”. Could you predict the view?